Prefer narration? Listen to the audio version of this post.
Introduction
NVIDIA’s market capitalization crosses the $2T mark. Sam Altman begins laying plans for a $7T global chip empire. The largest sovereign wealth funds compete to buy FTX’s Anthropic stake.
It’s easy to think the AI world is headed in one direction - bigger, badder and faster.
But in the last few months, we’ve heard of multiple generative AI and infrastructure companies stockpiling expensive GPUs, before struggling to find a use for them. Start-ups we know have been removing powerful frontier models from their tech stacks, in favor of small, open-source alternatives. And, of course, there are the fresh reports of frontier model providers that are struggling with revenue growth and margins.
This isn’t because ‘deep learning has hit a wall’ or that the technology is anything other than incredibly impressive. Instead, we believe that the following features can all be true:
the capabilities of large-scale AI systems have improved rapidly,
eye watering capex investments to drive 1) continues apace,
the exact shape of many commercial applications remains unclear,
while the AI sector itself remains in its early innings,
and the economics of large AI models don’t currently work.
There are also specific dynamics at play in the frontier model space that will likely prevent the market from correcting in the near future. These have the potential to impact the entire AI value chain, from the chipmakers through to investors and founders.
2014 SaaS vs 2024 jumbo jets
The meteoric rise of AI-related VC investment that started in 2014/15 was primarily driven by start-ups applying deep learning to well-established pain points for enterprise. These companies had low capex needs, namely some laptops and a cloud service. While it took 1-2 years to collect labelled data to build and train a model and move to a product, it was possible for relatively lean teams to have an outsized impact, while spending what we’d now consider small sums of money. These companies also maintained stable and predictable unit economics as they scaled. While they made less general purpose models, they made up for it with palatable economics and were often able to raise at generous valuations that offered them several paths to ultimately generating returns for themselves and investors.
When we enter the frontier model world, however, we see valuations that ordinarily would only make sense for a company that had invented a literal, rather than a metaphorical, money printer. To take some recent examples:
Elon Musk’s xAI is allegedly in talks to raise $3B at an $18B valuation, despite the company not having firmed up a clear business model;
Holistic, started by former DeepMinders, is raising a €200M maiden round;
Before being hollowed out by recent events, Inflection had raised $1.5B at a $4B valuation, despite revenue in the low millions.
Even in the calm waters of SaaS, eyebrows would be raised at these kinds of multiples. In fact, frontier model providers are operating in a space that couldn’t be designed to make it harder to earn money predictably.
Firstly, there’s the obviously significant capex required to build a model.
While Sam Altman has suggested that GPT-4 cost a mere $100M to train, this is only true in a very narrow sense. It’s unlikely that anyone reading this post, if handed $100M, could go away and create an equally capable model, unless they have already invested significantly in previous models, projects, and infrastructure that could be repurposed. The true cost is orders of magnitude higher.
Secondly, the unit economics remain messy. At the moment, providers are all losing money on their models, with growing revenues making little dent in compute costs and R&D expenses. However, SaaS economies of scale don’t apply here - growth in adoption does not necessarily mean the relative cost of infrastructure decreases.
Additional users, especially if they opt to use the largest, most resource-intensive models, can significantly increase the compute requirement. While there are efficiency gains from optimally using GPU resources, these plateau after a certain point. For a company charging users a fixed rate per API call or by usage tier, this becomes a tightrope walk.
When we start digging into the numbers, it gets ugly quickly. If we take Anthropic as an example, after paying for customer support and servers, its gross margin works out in the 50-55% range (versus an average of 77% for cloud software). Of course, gross margin does not reflect the cost of model training or R&D. It’s currently unclear what catalyst would change these margins radically.
The economics of open source pose their own challenges.
While the new Databricks model DBRX cost $10M to build and Llama 2 is thought to have cost $20M, the same caveats about carrying over investments apply. As costs inevitably grow with model size, very few organizations or individuals are likely to be prepared to pump ever larger sums of money into something that is ultimately freely available.
Open source tools can sometimes create a ‘lock-in’ effect, but it’s not apparent that the same will necessarily happen with open frontier models. If we take another Meta open source offering, React, which is used for building user interfaces, it’s easy to see why switching can be challenging. Once developers build applications around React, the team’s skills, tooling, and codebase become tied to it. Switching to another UI framework becomes a significant logistical pain point that could also involve changing the composition of a team.
Meanwhile, switching LLMs is a more straightforward process. It usually involves updates to specific features and components that directly interact with the model, which are comparatively isolated, and then running relevant benchmarks to ensure behaviors are consistent. The process becomes easier still for API-only models.
Given the gradual convergence in performance we’re seeing between different frontier models for economically useful tasks, this poses a challenge to the entire sector. We’re slowly entering into a capex intensive arms race to produce progressively bigger models with ever smaller relative performance advantages.
The airliners of the technology world?
This combination of a relatively undifferentiated offering and high capex before earning a cent of revenue is highly unusual for software. The IBM System/360 mainframe, which cost $5B in today’s money, is likely the only precedent. However, it marked the birth of a 50-year long hardware and software lock-in for the company. For frontier model providers, these trends are only likely to become more unfavorable.
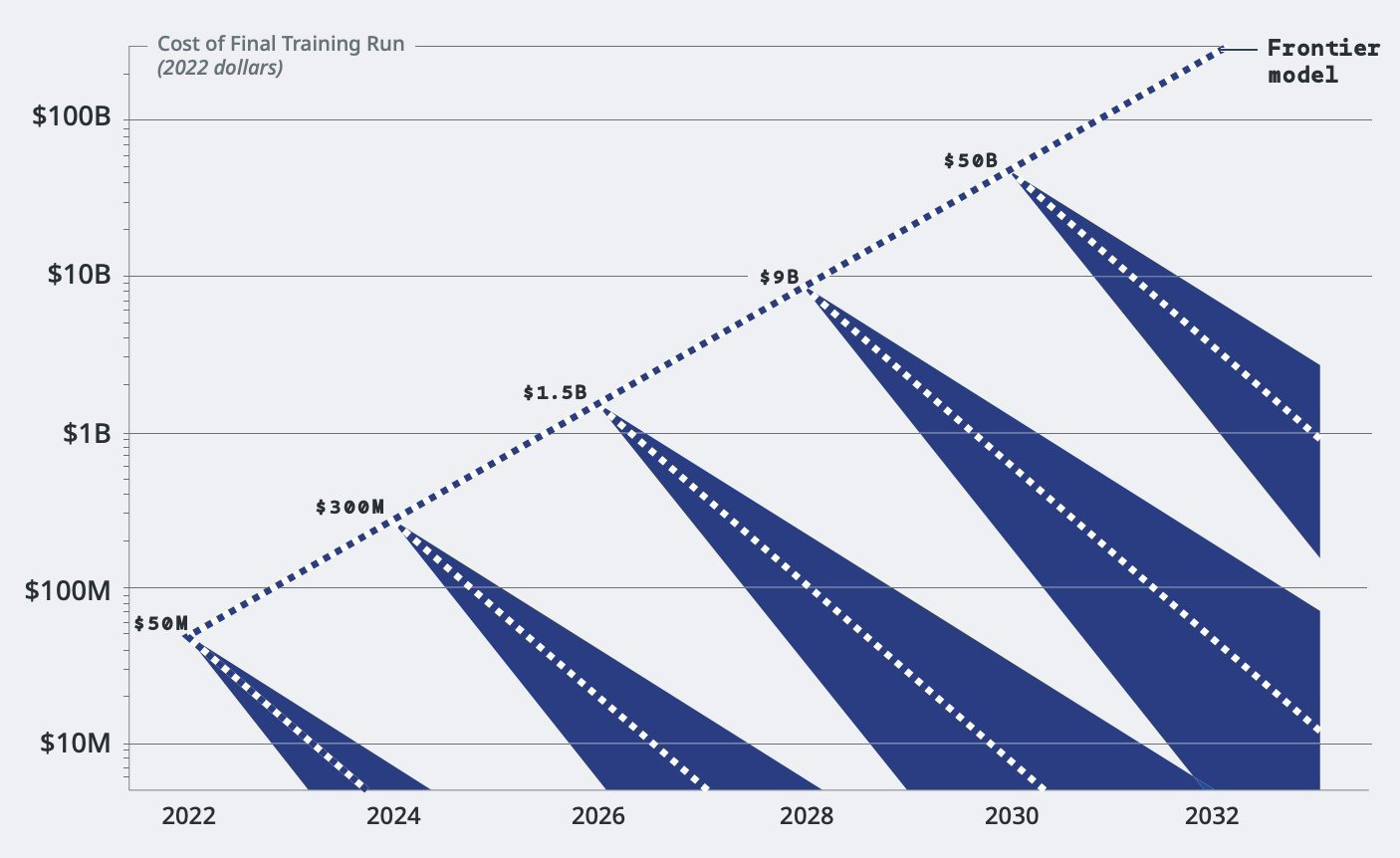
Warren Buffett warned that “the worst sort of business is the one that grows rapidly, requires significant capital to engender the growth, and then earns little or no money”, one where “investors have poured money into a bottomless pit, attracted by growth when they should have been repelled by it”. He was, of course, talking about airliners.
Unfortunately, airline operators have certain levers at their disposal that frontier model builders lack.
Firstly, airlines drive as much efficiency as possible in their service, eroding the customer experience as they try to eke out individual planes for 20 to 30 years.
Secondly, the industry engages in periodic bouts of suicidal price warfare to increase market share at the expense of margin - much to the chagrin of institutional investors.
However, foundation model providers don’t currently have the first option available to them, with the exception of persistent rate-limiting.
They struggle to eke models out for 20-30 weeks, let alone years. The economic value of legacy models can plummet within months of their release.
This is stark when you look at OpenAI’s GPT pricing options:
Meanwhile, there’s a parallel war playing out in API pricing, driving the cost of serving some new models down to essentially zero - sometimes within days of launch:
This turns the market into a competition to raise as much money as possible from deep-pocketed big tech companies and investors to, in turn, incinerate in the pursuit of market and mind share.
In industries like this, where the offering is similar and the economics are rough, consolidation is the norm. Historically it occurred across airlines, the automotive industry, telecoms, along with banking and financial services. Considering the compute and data requirements, the narrow talent pool, and the need to price sustainably, the frontier model space should be ripe for this.
However, regulators on both sides of the Atlantic have all but guaranteed that this is not going to happen. Something as trivial as Microsoft’s $15M non-equity investment in Mistral is considered worthy of a competition investigation by the European Commission, while the FTC is already probing the relationships between a number of labs and big tech companies. Even innocuous data licensing deals are being placed under the microscope. This is the climate that produced the bizarre pseudo-acquisition of Inflection by Microsoft.
But what about the customers?
So far, we’ve focused on the challenges faced by the providers of foundation models, but this is only part of the picture. Adoption of large models relies on users seeing economic upside that justifies the costs involved, whether that’s through API access or hosting.
Hosting a large model can quickly become prohibitively expensive. For example, a Falcon 180B deployment on AWS, using the cheapest recommended instance, will work out at approximately $33 an hour or $23,000 a month. This stands in contrast to a Falcon 7B deployment, which will set you back $1.20 an hour.
It’s harder to forecast prices for closed models, as there will be significant variation in token requirements and there can be multiple hidden costs (e.g. background API calls for different libraries and frameworks). Experimenting with different pricing tools shows how the numbers can begin to add up very quickly, even while remaining loss-making for the model builders.
Small is beautiful
Currently, we believe most economically useful foundation model applications do not require high powered multi-trillion parameter frontier models. The reason why many applications have struggled in our view is less about whether a model scores 82 or 86 on MMLU, but in how they’re applied.
Recently, Shyam Sankar, the CTO of Palantir, wrote a searing critique of the “cargo cult” mentality in software. He argued that companies focused far more on the process of acquiring technology than on deeply understanding or integrating it into their real-world operations. As decision-makers get caught up in layers of abstraction, software solutions become disconnected from the measurable outcomes they’re meant to be driving.
In the context of foundation models, this usually results in either the ineffectual layering of LLMs onto decrepit software stacks or the inappropriate use of off-the-shelf models with little to no fine-tuning.
As well as education levels increasing, there will likely be potential technical fixes. Our friends at Interloom are already redefining process automation, while longer-term, we can see context length playing a role. For example, there are already promising experiments that use massive custom prompts (e.g. a company’s code base or documentation, along with better prompt engineering) as an alternative to either fine-tuning on knowledge bases or RAG.
It could be that a world of smaller models with long context lengths end up becoming the go-to for the vast majority of common applications. Smaller models, in the 7B range, are compatible with a broad spectrum of GPUs, including the older V100 and A100, which are less powerful, but more cost efficient than the newer H100. The Apple M-series chip, with its integrated CPU/GPU unified memory architecture, means it’s already possible to run Mistral-7B on some Macs.
We can already see the future seeds of this being sewn. Google’s lightweight CodeGemma family is available openly in 2B and 7B form, while Haiku, the smallest of the new Claude 3 family, offers 1 million input tokens for only $0.25. Despite likely being an approximately 7B parameter model, it surpasses the 2023 version of GPT-4 (a likely trillion parameter model) in the LMSYS leaderboard.
Alternatively, we’ve written before about the benefits of open source models for innovators, including control, predictability, and removing reliance on a single external point of failure.
While this work is still in its early stages and has its skeptics, a future shift to on-device deployment, whether on a high-end desktop computer or powerful phone, is already beginning to take place. Microsoft Research’s BitNet paper outlined a language model architecture that uses very low-precision 1-bit weights and 8-bit activations, instead of the typical high-precision weights employed by most LLMs.
This produced significant efficiencies in memory usage and energy consumption. The researchers demonstrated that BitNet could maintain competitive performance on language tasks, while providing substantial reductions in the compute and power requirements. It was also able to mimic the scaling laws of high-precision models while maintaining the same efficiency benefits. We’re already beginning to see others train models using the same method.
Closing thoughts
The shift to on-device will be a slow one. Generalizing this work to domains beyond language and figuring out some of the complexities around real-world deployment and engineering is complex. We’ve also not entered the aggressive system optimisation era.
That said, there is cause to be optimistic - often models used for image generation or speech recognition and text-to-speech are smaller than text-generation models. While they require more computational power, this is easier to scale on-device than memory capacity or bandwidth.
If we one day moved to on-device hosting for most commercial applications of generative models, the implications could be seismic. We could see demand for high-end GPUs shrink significantly, a market open up for edge chips, while VC firms would contribute less to the bottom-line of cloud providers.
While highly powerful frontier models would remain available for the small subset of users who need their advanced capabilities, others could redirect investment away from ever higher cloud bills to more productive ends. It would also end the unhealthy dynamic of equity being used for capex and the aggressive dilution of founders and early investors to pay GPU bills.
Of course, the economics of this space remain in flux. The potential of the largest frontier models to develop agentic capabilities and take on more autonomous decision-making and problem-solving may lead to a re-evaluation of their economic value. But we suspect that, for the majority of commercial applications, end customers will still index on predictability and reliability over unbounded autonomy for the foreseeable future. A sudden advance in capabilities also does not magic away upfront capex or operational costs; if anything, it likely worsens them.
We’re unashamedly optimistic about the potential of AI to transform the economy and believe that industries will be rebuilt around it. None of this is an attempt to pour cold water on frontier models or the progress of recent years. Instead, it’s an acknowledgement of a simultaneously obvious but often forgotten reality: that companies eventually need to make money.
This means that we believe that we’ll increasingly see a split in the AI world: between i) predominantly closed frontier models charging economically viable prices to a small subset of deep-pocketed users that need their capabilities ii) efficient, cheaper open source models with a specific task focus. This diversity of providers is the ideal outcome for the ecosystem, but it’ll only happen if it’s allowed to. Whether it’s on safety or competition, badly designed regulation poses the biggest threat to the future function of the market. If we get this wrong, a future of decaying frontier AI airliners awaits us.
Very good read, thanks for this.
Good analysis