California’s AI bill was an avoidable disaster
What went wrong and how to prevent this from happening again
Prefer audio? Listen to this article here
Introduction
At Air Street, we believe that breakthrough moments in technology aren’t just the product of great work. There’s a set of political, institutional, and economic factors that need to line up as well. We saw this up close when we campaigned successfully on university spinout reform with our Spinout.fyi work. Great research and proto-companies were being smothered at birth by bad policies that made it impossible for founders to raise money. It’s why we urge founders to think about these questions from day one.
As we covered in the most recent Guide to AI, we’ve been watching recent developments in California with growing alarm, as the state lines up to pass SB-1047. Sponsored by the x-risk focused Center for AI Safety, it aims to introduce a safety and liability regime for foundation models. If passed, we worry that not only will it have a negative impact locally, other jurisdictions will seek to imitate it.
We believe that supporters of innovation and progress have been routinely outclassed in policy fights through a series of unforced errors. These battles for open source are too important to keep losing. This means working significantly harder, digging deep into our opponents’ arguments, and learning from their successes.
Why is this bill bad?
In short, we think this bill is badly drafted, likely to drive up costs for developers, increase the centralization of the AI industry, and damage open source research.
We see this, firstly, in how the bill is scoped. The bill covers any model that “was trained using a quantity of computing power sufficiently large that it could reasonably be expected to have similar or greater performance as an artificial intelligence model trained using a quantity of computing power greater than 10^26 integer of floating point operations in 2024”.
Compute and performance are not the same thing and as Rohan Pandey of ReworkdAI has argued, it could easily result in the threshold being set arbitrarily low if someone releases a suboptimally trained model. There can be good reasons for suboptimal models (e.g. Llama 3, so it could be deployed on easily available hardware) or bad ones (e.g. a big tech company deliberately inflicting low thresholds on competitors).
The bill also poses a serious threat to open source. Despite the bill containing provisions for a new advisory council to advocate for open source, it will have the reverse effect. Not only will developers be tied up with extensive reporting and compliance requirements, they face a challenging liability regime that will make them responsible for misuse of their models. They’re also forced to prove a series of negatives through the compliance regime - e.g. that there is no way a model could make it easier to do things that cause $500M in damage via multiple related incidents. Not only is this essentially impossible to quantify, it’s a standard that search engines and many well-stocked libraries would struggle to meet.
When your opponents are serious…
When you look at the x-risk discourse of last year, it’s easy to assume that proponents of safety legislation were primarily the lucky beneficiaries of blind panic. As luminaries from the technology world lined up to say there was a real risk of extinction or other catastrophic harms, they benefited from a rush to legislate. But this isn’t really the case.
Fear might have raised interest in the subject of AI regulation, but it didn’t spawn concrete proposals. After all, when faced with a breakthrough like LLMs, many policymakers would:
Struggle to conceptualize the exact shape harm could take - extinction is abstract and they might not jump to issues like biosecurity unaided;
Be unsure about to approach regulating it;
Be keen not to lose out on any economic benefits of the technology.
It’s true that they were lucky in the sense that legislators and regulators like to legislate and regulate. There’s an innate bias towards action - it’s usually easier to persuade them that they should do something rather than nothing.
Safety organizations have mastered the art of producing policy recommendations that are simultaneously radical, while making them sound technocratic and conservative. In fact, they will often tell policymakers that their proposed recommendations will only affect a tiny fraction of models. This is often technically true, but misleading. As the talented, but anonymous, 1a3orn has pointed out - many of these organizations, including the Center for AI Safety, have said versions of this, while essentially wanting the de facto ban of the release of significant numbers of open source LLMs, including Llama 2.

A regulation that banned any foundation more powerful than Llama 2 will technically only regulate a tiny fraction of all AI models everywhere, but it will regulate a significant proportion of the capable foundation models researchers and enterprises actually use. They’re just hoping an uninformed audience won’t spot this sleight of hand.
This allows them to make the problem seem tractable (which regulators like) and to avoid the appearance that they’re likely to have much economic impact (which politicians like). The proposals are rarely scrutinized by people able to contextualize FLOPS limits or question the eccentric use of MMLU scores as a measure of safety. Advocates of safety legislation know they’re on safe ground attacking Big Tech, but are aware that there is more residual warmth towards open source, so will usually say some warm words about its importance, while subtly undercutting it. The California bill is a prime example of this.
Beyond skillfully presenting well-chosen objectives, safety advocates also present credibly to policymakers. In part, this is because many luminaries in the AI world do genuinely agree with them.
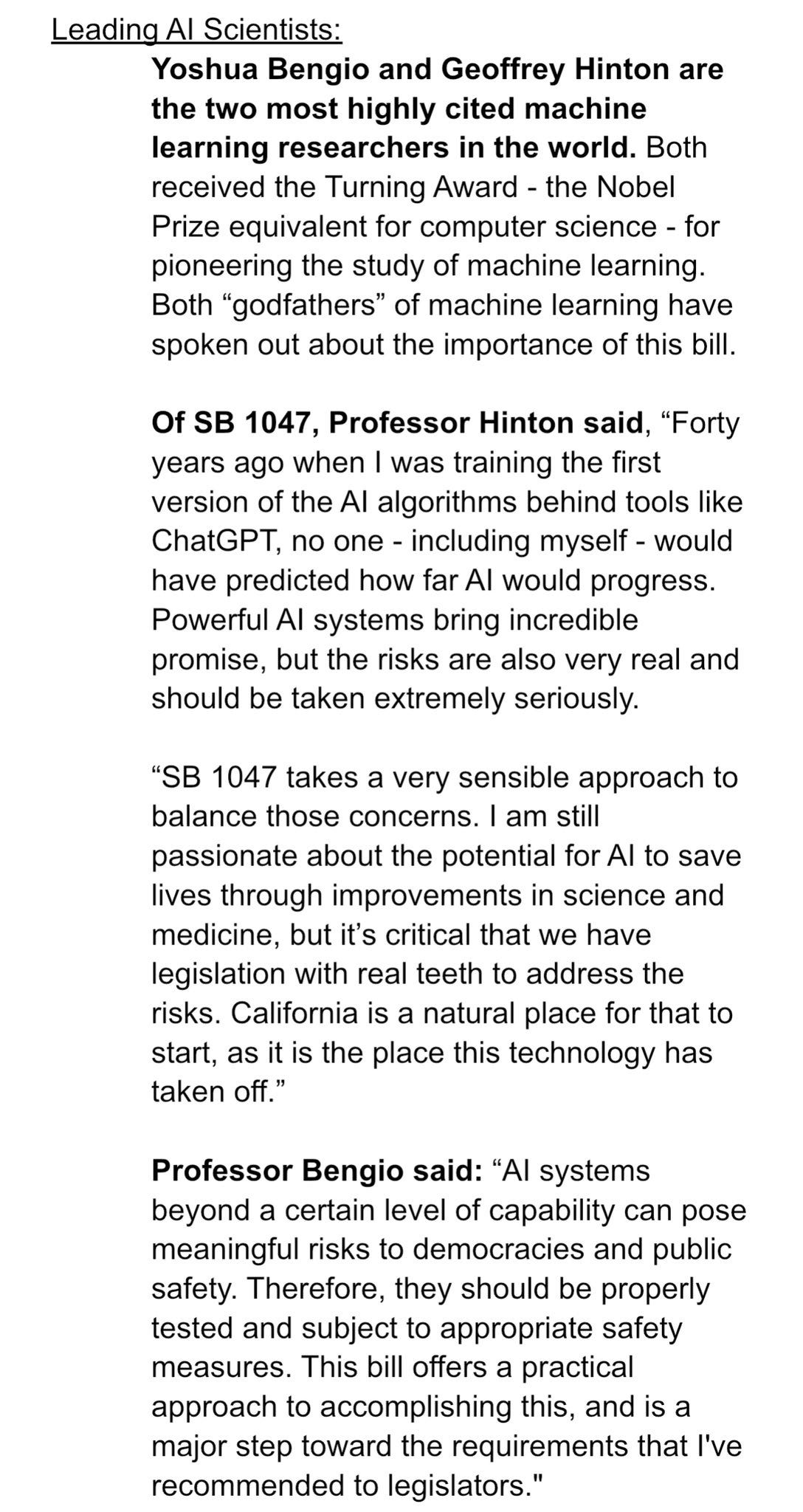
These famous names might be a minority of practitioners and include people who are no longer working at the frontier, but they’re hard to dismiss. But more importantly, they’ve put the work into creating a network of think tanks and campaigning groups that do a very good job at not looking like lobbying groups. They have neutral names, produce research, and design explanatory material with accompanying graphics. This isn’t proof of a cabal or a conspiracy - it’s good public affairs work.
…you need to respond seriously
The undoubted skill of the bill’s proponents is one half of the story. The other is the failure of the opponents of regulation to formulate a compelling counter-narrative. Opponents of AI safety-focused legislation have made two critical errors over the past year: doubling down on loser arguments and focusing their energies on X rather than direct engagement.
Let’s take these in turn.
Loser arguments
Opponents of AI safety-focused legislation have failed to undercut the pillars of the safety-ist case, opting for a set of lines that are either overstated or unlikely to persuade their target audience.
Probably the most prominent rallying point was “regulatory capture” (RC) - the concern that Big Tech would take advantage of the panic to impose expensive regulation that would kill off open source and drive up compliance costs for challengers.
To be clear, RC is real and it has a long, dishonorable history. It is inevitable that big companies will attempt it whenever they can. There are bad faith actors in the x-risk debate, stoking the panic for fundraising or commercial purposes. And of course, when politicians out and out ask CEOs with a vested interest how their industry should be regulated, it’s important to be vigilant.
However, in the past few years, Big Tech has largely failed to block or even meaningfully shape the regulation that affects it. Take GDPR. The legislation may technically provide incumbents with some advantages over smaller competitors, but if it’d had the power, Big Tech would have killed it. The theoretical competitive benefits probably aren’t worth the permanently higher legal bills, risks of sanction (which have run into the billions by this point), and inferior product experiences. The intensive lobbying was about damage limitation more than anything.
Even if its accuracy was unassailable, RC suffers from three other problems.
Firstly, it’s too complicated. The pro-regulation case is straightforward, emotive, and intuitive - “this is moving very quickly, it’s scary, we should include some checks and balances”. RC requires you to explain industry dynamics to someone unfamiliar, before you then explain the downsides of particular intervention. It’s too long and complicated to capture the attention spans of busy people. That may well be a depressing reflection on how policy gets made, but we quite literally don’t make the rules.
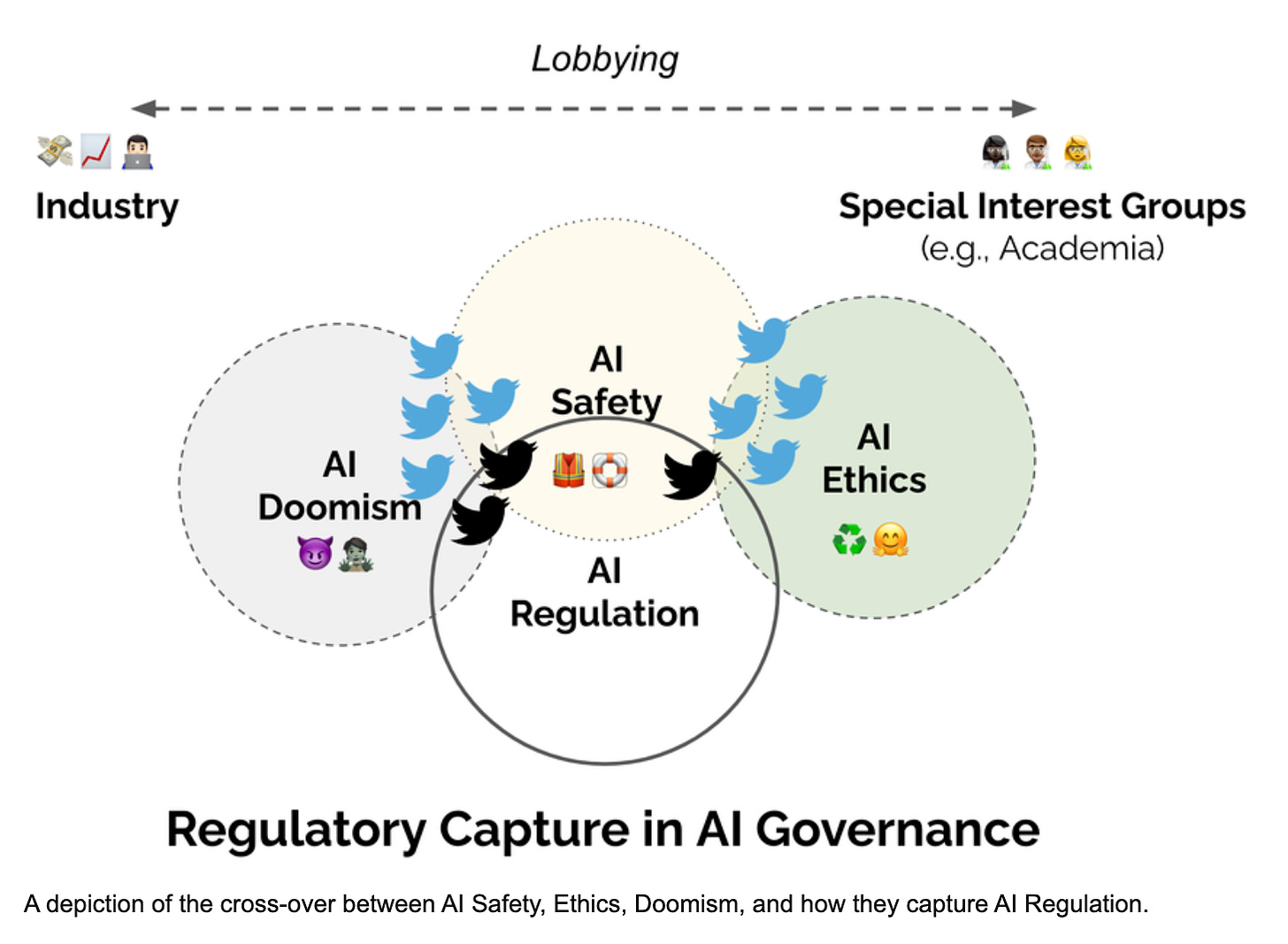
Secondly, even if the audience understands it, it’s unlikely to resonate. Attacking your opponents’ motivations is an easy source of likes and retweets, but it doesn’t land with policymakers. Trying to tell a pro-regulation policymaker they’ve been duped by their allies is a recipe to get dismissed as a conspiracy theorist. Similarly, regulators will balk at the suggestion that they either have been captured or would be susceptible to it.
Finally, it doesn’t work as a catch-all explanation. Not all proposals for AI regulation are the same. You can reasonably argue that last year’s White House executive order had Big Tech’s finger prints on it. However, while Big Tech companies may benefit relatively from the current California bill, they didn’t actually lobby for it. You instead have to start launching into an explainer of online rationalism and effective altruism to regulators. Good luck with that.
The other popular argument that gained traction is the notion that regulating the technology itself rather than at the application level is innately wrong. We shouldn’t ban gradient descent, we should regulate bad applications.
This is an improvement on regulatory capture, because it’s:
True to an extent
Snappy
Not an attack on people’s motives
Proposes an alternative theory about how we should approach these questions.
Unfortunately, it isn’t as robust as it sounds once you attempt to generalize the logic beyond AI. Large numbers of foundational tools or technologies are regulated at a technology rather than an application level. We see this in everything from radio frequencies through to internal combustion engines and encryption standards.
X over direct engagement
The shaky substance of these arguments can be traced back to where the opponents of this legislation have staked out their ground: X. On one level, it’s understandable - it’s where the AI community lives. Unfortunately, it’s not where the people who will be regulating the AI community spend any time at all.
The dynamic on X is unhelpful for policy engagement for a few reasons:
It can serve as displacement activity - you feel like you’re having an impact on the policy conversation, even though you usually aren’t;
It primarily exposes you to the concerns of people you agree with, rather than the people you need to persuade, which means reinforcing each other as opposed to battle testing arguments;
You usually talk to people with an extensive background in your field, so you lose the discipline of making your case clearly to outsiders or newcomers;
You rarely have to engage with your most sophisticated opponents or the best versions of their arguments, which breeds laziness.
It also means that real-world engagement is left to a relatively small pool of people or organizations, whose power over the debate is limited. They are either individual researchers or start-ups, who have limited reach. Or they’re corporations like Meta and their industry coalitions, who are largely mistrusted by policymakers.
This means there’s just no equivalent to the ecosystem of organizations producing research, policy proposals, or educational materials. One side has just not shown up to the fight.
The other big downside of an X-bias in engagement is that it encourages an overconfident tone that policymakers find off-putting. The e/acc spirit is good motivation for some builders, but to a political class that’s terrified about AI, “scream if you want to go faster” is the wrong message. Against a wave of scientific-looking papers and the luminaries of the AI world, opponents of regulation are choosing to fight back with memes and AI-generated images of futuristic cities.
What should we do instead?
While the safety advocates have a lead, there are some reasons for hope. First of all, it is possible to ‘win’, provided you have a realistic goal. You aren’t going to stop all AI safety regulation everywhere in the world - there is no precedent for this in the history of technology. But it is possible to mitigate the worst of these policies. For example, following heavy lobbying from Mistral, the EU ended up modifying some of the worst provisions around open source foundation models from the AI Act. The company had hired a former French digital minister as an advisor, and heavily campaigned on competitiveness and the threat over-regulation posed to small businesses.
So, how should skeptics of California-style legislation approach these issues? We have a few suggestions:
Stop discussing the motivations of safety advocates. Analyze the materials and research being shared by safety advocates and produce a reasoned rebuttal. Proceed as if they are acting in good faith. Treat their most sophisticated arguments seriously, even if you don’t believe they are serious. 1a3orn’s methodical rebuttal of bad AI biorisk literature is a great example.
Don’t waste time arguing with the most extreme voices. While people calling for a total pause or halt to AI development are prolific on X, they are marginal in the real-world and are not persuadable. No one is going to bomb GPU clusters.
Gather data or examples, put a human face on it. Bad AI regulation would be catastrophic for start-ups. When we were working on spinout reform, deconstructing the bad arguments universities made was part of the story. The other half was collecting data and experiences from hundreds of founders with first hand experience of the dysfunctional system. This was crucial for convincing policymakers that this was a real challenge.
Move beyond X. While X is a great tool for gathering information, finding allies, and sharing arguments with your supporters, it’s a bad vehicle for persuasion in policy fights. Individual companies like Mistral will be better-placed to run on-the-ground lobbying operations; there's nothing to stop the community producing its own hubs of easily accessible materials, op-eds, or reports. With spinouts, we found that while these perform less well on social material, they’re the material that more process-driven policymakers actually read and remember.
Make a tangible case. Too many anti-regulation arguments rely on abstract appeals to progress or acceleration. These either don’t register with policymakers or provoke fear. What’s an example of important research that wouldn’t be possible with a piece of legislation? How would a start-up founder’s life be more difficult?
Develop alternative policy proposals. Jumping back to the beginning - legislators are dispositionally biased towards action. Arguing that they should do literally nothing in the face of a wave of technological change is a doomed proposition. Whether it’s building regulatory capacity, as our friends at Form Ventures have argued for, sensibly clarifying tricky legal questions (e.g. liability), or focusing on high risk applications where existing regulation is inadequate, there are useful things policymakers can be doing.
Closing thoughts
While these fights play out, we don’t plan to relax and backseat drive. We will continue to advocate forcefully for policies that support founders and call out bad legislation when we see it. We’re also open to supporting any community efforts to rebalance the debate. If you’re interested in potentially working together on any of these issues, don’t hesitate to get in touch.
Interesting. However two comments :
A) you say "Why is this bill bad?", but you don't answer the opposite question, in what it's good, or present alternatives. B) something imperfect that can be improved on is better than nothing.