The State of AI Report aims to provide a comprehensive overview of everything you need to know across AI research, industry, politics, and safety. To ensure the report remains at a manageable length, lots of material doesn’t make it into the final version. We’re bringing Air Street Press some of the research that didn’t make the original cut, along with our own reflections.
Introduction
This year’s State of AI Report contained our largest ever embodied AI section, covering everything from diffusion models through to open source robotics libraries, humanoids, and self-driving. Until relatively recently, with the partial exception of self-driving, embodied AI was the unloved cousin of AI research.
Work in this field, which concerns the symbiosis of software and hardware to enable AI to run onboard of physical machines and control their function, was concentrated in a small, dedicated community. It was rarely more than a side-project for the biggest labs. But a combination of dedicated entrepreneurship and progress spurred by the creative application of foundation models has spurred a renaissance.
Robotics: a GPT moment?
One of the biggest stories of the year is the transformation of robotics by foundation models. LLMs are replacing hand-coded robot behaviors with natural language commands that robots can flexibly execute, while VLMs enable powerful scene understanding that unlocks rapid learning of new behaviors. Together, they can be used to generate diverse training scenarios and allow robots to generalize across tasks without custom policies and reward functions for every situation.
By making it possible to control robots via natural language, rather than via cumbersome proprietary software and abstruse programming languages, LLMs have significantly improved the accessibility of robotics. For example, our friends at Sereact were the first to combine zero-shot visual reasoning with natural language instructions to create PickGPT, software for human operators to interact with robotic arms in warehouse settings.
In our report, we’ve noted the steady drumbeat of foundation models, frameworks and datasets emerging from Google DeepMind and other labs. Now all believers in the robotics future, they’re keen to occupy as much of the stack as they possibly can without building the hardware.
Back in February, NVIDIA established its GEAR (Generalist Embodied Agent Research) group under Jim Fan and Yuke Zhu. The two had previously cooperated on related projects, most notably Voyager, an LLM-powered agent in Minecraft. GEAR research includes humanoid foundation model Project GR00T and imitation learning method MimicPlay.Â
NVIDIA is already working with a bunch of humanoid start-ups, including 1X Technologies, Agility Robotics, Apptronik, Boston Dynamics, Figure AI, Fourier Intelligence, Sanctuary AI, Unitree Robotics, and XPENG Robotics. This is a classic NVIDIA move and one they used with self-driving: work every potential partner, regardless of their success prospects, using the learnings to build your own autonomy stack.
Meta has also been making moves in this space. Back in January, they released OK-Robot, an open framework that enables robots to perform pick-and-place tasks in new and unstructured environments, such as homes, without needing prior training.
In our most recent edition of Guide to AI, we covered a major drop of Meta research that focused on touch perception, dexterity, and human-robot interaction in a clear pitch for the humanoid marketplace.Â
Humanoids are also the center of OpenAI’s robotics efforts. The company has invested in Figure AI, 1X Technologies, and Physical Intelligence and its new robotics team is collaborating with external partners rather than attempting to compete against them.
So what does the humanoid market opportunity look like?
As the heading for this slide suggests - we’re not entirely convinced. While companies routinely release slick-looking demo videos, actual real-world performance is lackluster. We were underwhelmed by the videos of the beer-serving Optimus at Tesla’s autonomy event which, as is often the case with humanoids, was not actually autonomous.Â
The promise of humanoids boils down to significant upfront capex, in exchange for something that works slower and less reliably than a human, while requiring maintenance and a cloud connection. The bet is that, in the end, the per-hour cost will work out more cheaply than human labor.Â
This may well end up working out for a handful of large companies prepared to make the investment, but does it have the potential to disrupt the more conventional industrial robotics market? Systems that combine off-the-shelf components with AI-powered software can already serve beer and fulfill most warehouse functions efficiently and cheaply.Â
Why the self-driving parallel?Â
Self-driving: the struggle to scale
In past instalments of the report, we covered how self-driving car companies would make extravagant promises about performance and testing, only then to fall short. This is beginning to change.
Waymo is now hitting its stride, but it took fifteen years, over ten billion dollars of investment, and some luck to get there. Cruise and Uber both suffered serious setbacks after pedestrian accidents, while Apple abandoned its self-driving efforts after $1B in investment. The physical world is hard and we suspect humanoid builders will discover this pretty quickly.Â
Our report also covered Wayve’s growing traction. The company’s journey from a £2.5M seed round in 2017 to a $1.05 billion Series C is a case study in both courage and capital efficiency. A fortnight after we published the report, Wayve announced that it had opened a new hub in San Francisco to accelerate its testing and find local partners.
The Wayve team will have been cheered by their competitors over at Waymo’s unveiling of EMMA, an end-to-end model for self-driving. Wayve were the original pioneers in end-to-end, which uses raw sensor data (like camera inputs) directly to control outputs.
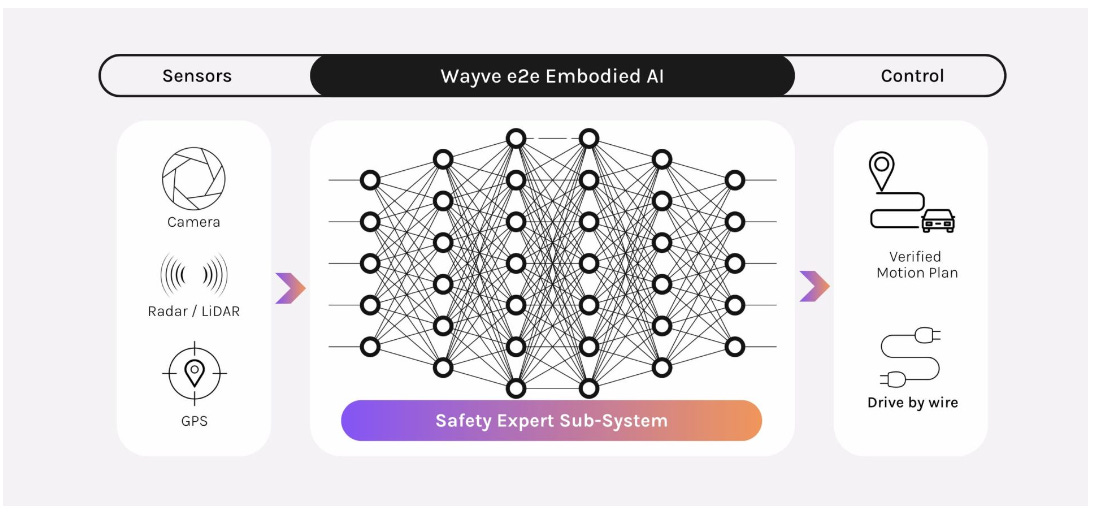
This has the advantage of simplicity, as it removes the possibility of errors building up in separate modules, and adaptability. Historically, most self-driving companies shunned end-to-end, believing it to be too data intensive and uninterpretable.Â
EMMA, built using Gemini, represents all inputs and outputs (such as navigation instructions, vehicle states, and planned trajectories) as natural language text. This allows EMMA to apply language processing to tasks traditionally segmented into modules, like perception, motion planning, and road graph estimation, effectively merging these functions. It also solves the interpretability issue, with driving rationale being expressed in natural language.
This tallies with earlier work from Wayve and serves as a reminder of how foundation models can strengthen generalization, robustness, and data efficiency outside the domains we normally associated with NLP.Â
Closing thoughts
From Nathan’s personal support for Wayve in its founding days through to Air Street’s more recent investment in Sereact, we’ve long seen the opportunity in embodied AI. While AI-first approaches are already having a significant impact on the domain of data, knowledge, and language, their potential physical impact remains largely untapped. We would be very surprised if this does not become a significant theme of our 2025 report.
Hi Nathan, I would like to more coverage of embodied AI developments in your news letter :-)