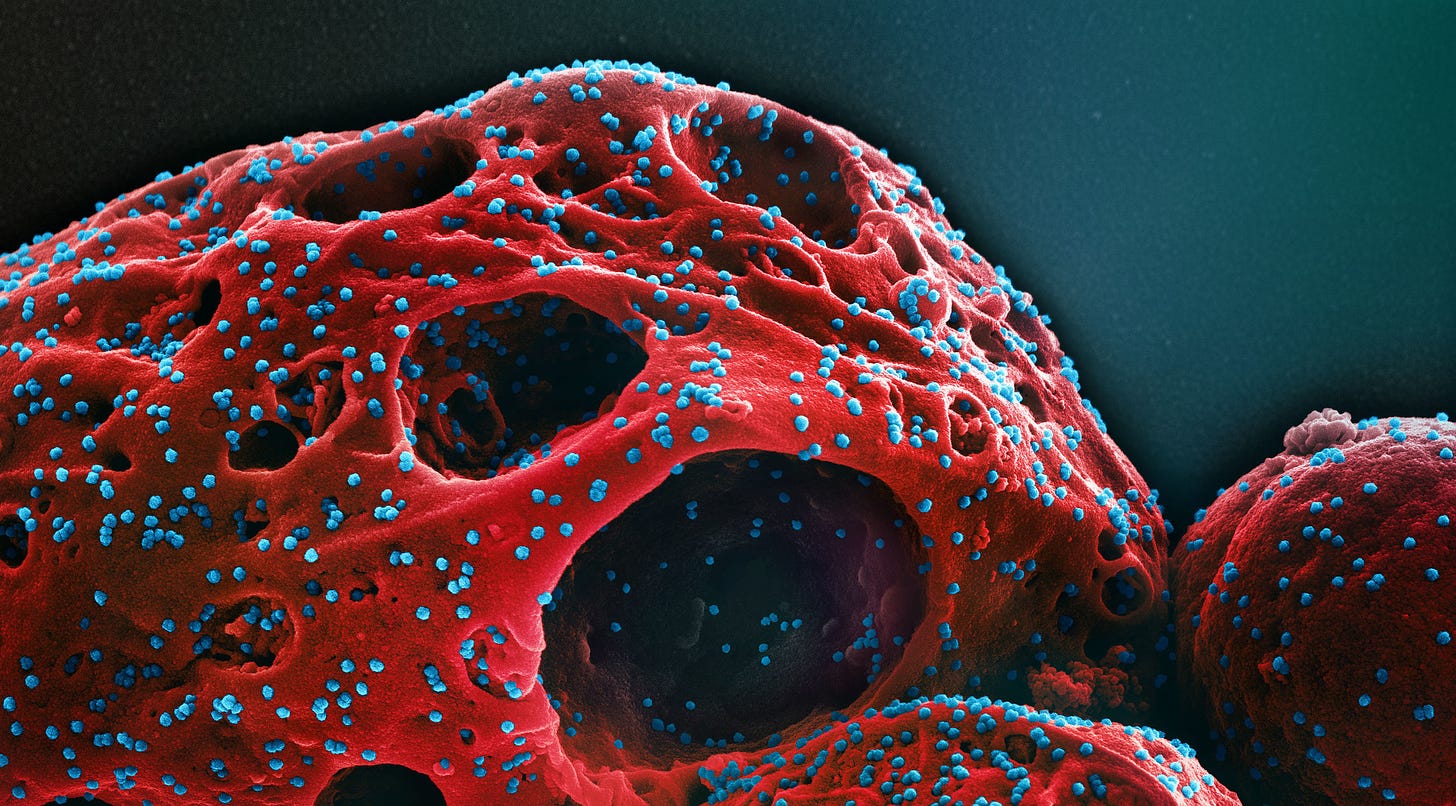
Introduction
Progress in AI-first biology has been stunning over the past few years. Traditional scientific exploration is being upended. Old-school methods are being ripped and replaced by modern deep learning approaches. For example, decades of work that went into cheating knowledge-based protein folding algorithms was swept away in fraction of that time by AlphaFold and RFdiffusion. AI-powered work on computational protein design is now considered Nobel Prize-worthy.
While the chorus of excitement for AI-first biology has grown, it is increasingly countered, not by AI naysayers (yes they’re still loud) but by biorisk-aware safety researchers. By biorisk, we mean the misuse of AI tools in a biological context, whether that’s to accelerate known bioweapon design (e.g. dangerous pathogens), develop novel bioweapons, reduce the barrier to creating such agents or to make them faster through lab automation.
We must first state our own priors: we’re highly optimistic about the potential of AI-first scientific research to do good in the world. For example, this year’s State of AI Report covered the work of our friends at Profluent in designing new open source genome editing enzymes that are active in human cells, which has the potential to accelerate life-changing medical research.
We see no reason to slow down this kind of well intentioned, therapeutically-important work nor do we think it should be over-regulated.
But technology is inherently dual use and not all actors are so well-meaning.
In theory, it’s possible that the AI-guided de novo design of biological and chemical agents, such as proteins, small molecules, and viruses, offers up biosecurity risks that are greater than we’ve seen up until this point.
Such novel agents could have properties that do not exist in nature, they could be more potent, or they could have unknown off-target effects. These risks are not entirely new in and of themselves, but the pace, potency and access of their development is what is potentially more worrying.
So this week we’re looking at how real of a risk biosecurity is in the age of AI-first design, whether current regulatory proposals are proportionate or likely to work, and some potential fruitful future inquiries.
The wrong risks?
The first wave of ‘biorisk’ panic has been primarily LLM-focused.
One of the key drivers to the AI risk panic that swept governments in 2023 was a study from Anthropic. Unpublished to this day, the research found that “unmitigated LLMs could accelerate a bad actor’s efforts to misuse biology relative to solely having internet access, and enable them to accomplish tasks they could not without an LLM”.
Around this time, there was a large volume of biorisk research funded by organizations like Open Philanthropy that began to gain traction. While we don’t know much about the design of the Anthropic research, much of this material seems weak. Sweeping significance was attached to the ability of LLMs to answer a handful of questions about pathogens, as opposed to their ability to help plan an attack, while little effort was made to assess their marginal risk versus internet access.
A number of organizations have tried and failed to replicate Anthropic’s results, including OpenAI and the RAND Corporation. Neither of them found any increase in marginal risk from the use of LLMs relative to internet access or other resources.
While OpenAI may have classified o1 as a ‘medium’ biorisk (a first for an OpenAI model), the company said that “the model cannot yet automate biological agentic tasks”. Although it performed significantly better than 4o on biothreat information questions, it performed poorly on actual ideation.
When it comes to the execution of biological attacks with known pathogenic agents, it’s unclear the extent to which text-based LLMs will ever pose a greater marginal risk versus existing tools.
Instinctively, it’s hard to imagine a plausible profile of an LLM-aided bio-attacker: someone with all the advanced lab skills, equipment, facilities and reagents required for implementing protocols (without killing themselves in the process), but who cannot discover them from papers, textbooks and/internet access alone.
But maybe our preconception is wrong. For us, to demonstrate a compelling risk, research needs to show that:
Either access to a lab is not a meaningful barrier for a motivated actor or that LLMs mitigate some of the need for it;
Models can actually help a motivated actor troubleshoot or solve problems as they try to follow the ’recipe’, as is frequently posited but rarely demonstrated;
Whether 1) and 2) being true will make motivated actors more inclined towards biological attacks.
We are not currently aware of any research in the public domain that shows this, but this of course may change.
Wrong risks, wrong regulations
Our skepticism about Llama‘s ability to unleash chaos on the world should not be interpreted as complacency about biorisk as a whole. In fact, there’s good reason to believe that an overfocus on LLMs could be actively unhelpful for the cause of advancing biorisk mitigations.
Researchers have understandably become alarmed about the misuse of specialist biological design tools (BDTs). BDTs, as the name suggests, are tools trained on biological data that can help with the design of proteins or other biological agents. Think AlphaFold, RFdiffusion, the ESM family of models, or AlphaMissense.
As mentioned earlier, these technologies are essentially dual-use. A BDT could be used to design a new vaccine. But it could also be used to break down the genome that encodes a pathogen into small enough pieces of DNA (called oligos) and bypass the screening protocols of DNA synthesis companies. Researchers have managed to do this before, without access to the current generation of BDTs.
The world is moving towards regulating frontier AI, but this legislation focuses heavily on model scale and compute resources - both of which are poor proxies for biorisk.
Researchers spanning a coalition of risk-focused organizations have outlined how BDTs risk falling through the cracks of current regulatory approaches and outline some examples of best practice.
This approach makes sense for traditional frontier AI labs. Only a handful of well-resourced actors can build frontier models (that, at least today, are generally large-scale), which creates a relatively small pool of actors to monitor. Also, the sheer energy and infrastructure footprint is pretty hard to conceal. On this, it’s worth checking out our recent piece on AI and energy, which dives into the energy demands of advanced AI systems and why approaches like geographically distributed training are likely to struggle, at least for the foreseeable future, absent breakthroughs.
BDTs, on the other hand, are typically optimized for handling specific experimental tasks. In biological design, the input space is often much more structured. For example, protein sequences are composed of 20 natural amino acids, and for machine learning purposes their structures can be represented in a lower-dimensional space (e.g. using specific residue features or sequence embeddings). This contrasts with LLMs, where the input consists of potentially very large vocabularies of tokens with complex semantic relationships.
This means that significantly smaller and less computationally intensive models can still be very capable.
You see this trend play out in BDT-related research. For example, EvolutionaryScale’s ESM models are trained on vast quantities of protein sequence data in order for them to learn patterns of amino acid co-occurrence that reflect evolutionary constraints. ESM3, the company’s most recent multi-modal model, was tested at a variety of model scales. Its largest hit 98B parameters, but only marginally outperformed the 650M parameter version of ESM2 on sequence-only tasks. While ESM3 might hover around the US government’s 10^23 FLOPS reporting requirement, other models may not.
The risk researchers above propose a comprehensive framework for governing BDTs, acknowledging their dual-use nature in enabling both beneficial research and potential misuse. The framework identifies 25 measures across six key areas:
Responsible development (e.g. expanded dual-use review and voluntary commitments),
Risk assessment (e.g. including model evaluations and red teaming),
Transparency (e.g. impact statements and vulnerability reporting),
Access management (e.g. implementing structured access and KYC protocols),
Cybersecurity (e.g. protecting databases and securing lab equipment),
Resilience investments (e.g. public compute infrastructure access).
While these measures would likely be worthwhile ways of preventing the synthesis of known pathogens, it’s less clear how well they’d work for de novo pathogens.
These agents by definition don't have close natural analogs, which could make them harder to detect through traditional sequence screening methods.
Complacency?
Unfortunately, there is less evidence that governments are taking biorisks seriously. To be clear, we don’t have any secret insight into how Western democracies are approaching biosecurity. But as government outsiders, there are good reasons to be concerned.
For a start, governments leave clues about what they do and don’t prioritize.
While scrutiny has rightly been applied to poor biosecurity in Chinese labs following the Covid outbreak, Western governments have little to be complacent about.
In the US, a shortlist of labs working with particularly lethal pathogens receive dedicated federal oversight, but other labs face a confusing patchwork of regulations. This leads to ‘invisible biolabs’, working on potentially highly dangerous research, with little to no government awareness of their work.
Other governments approach biosecurity as an irritating cost. For example, the UK government created a successful waste water monitoring system in 2020 during the Covid pandemic, which it ceased to operate in 2022 as a money-saving measure. One former official we spoke to said the UK’s preference was to simply view biosecurity as something the World Health Organisation should sort out.
But wherever you are in the world, there is good reason to be concerned. In 2021, the Global Health Security Index scored only a quarter of the countries playing host to the 59 BSL4 labs (those working on the most dangerous pathogens) highly on safety.
So, should we be panicking?
We take a cool-headed view over at Air Street Press. Instinctively, we’re skeptical that non-state actors will find it easy to use these tools to effect large-scale harms in the world. There is already a decent volume of publicly-available evidence of how terrorist groups, including al-Qaeda, have dabbled in bioweapons.
But one of the most revelatory accounts we found in our research for this essay covered how Aum Shinrikyom, the Japanese cult that used the chemical agent sarin to carry out a deadly attack on the Tokyo subway in 1995, previously had a biological weapons program.
The program was led by a trained biologist, who attempted to manufacture both botulinum toxin and anthrax at scale. For the former, they bought up significant quantities of land and built drum fermenters. For the latter, a sympathizer who worked at a university likely stole an anthrax strain from a well-stocked lab.
Thankfully, this work wasn’t successful for a few reasons. The program leader lacked expertise in microbiology, so the team struggled to maintain the anaerobic conditions for botulinum production. They also likely failed to obtain sufficiently virulent strains. For example, the group only had the vaccine strain of anthrax to work with, while they struggled to find natural sources of botulinum bacteria with adequate toxin production.
What’s more, the equipment they used to try to spray biological agents frequently malfunctioned.
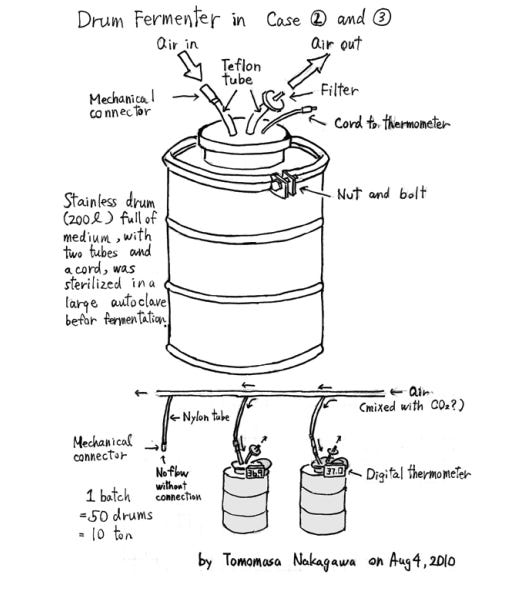
It’s theoretically possible to imagine AI helping to identify or engineer virulent strains more rapidly, overcoming the initial hurdle of wild-type pathogen efficacy. With the right AI design tools, datasets, reagents, lab equipment and facilities, for example, an actor could input the genome of a benign anthrax strain to identify the modifications necessary to produce more lethal strains. But to then implement this would be very challenging and - bluntly - there are easier ways to effect large-scale harm: build a bomb.
As Margaret Kosal of the Georgia Institute of Technology put it: “While such AI tools may lower barriers to entry for an extremely motivated actor, like in a sophisticated state-based program, to design highly toxic compounds in silico, using the databases of hypothetical compounds to actually create the chemicals, never mind weaponize them, still also requires sophisticated chemistry and engineering expertise and materials to synthesize the candidate compounds and to handle them safely until deployed. This is a difficult task for known chemicals, but one which becomes more difficult for novel chemicals whose properties have not yet been characterized or studied.”
Unfortunately for us, there are sophisticated state-based programs.
For example, North Korea unquestionably has science and tech talent and a relaxed attitude to targeting western institutions. The US State Department has concluded that North Korea “has a dedicated, national level offensive biological weapons (BW) program” and “the technical capability to produce bacteria, viruses, and toxins that could be used as BW agents” and “the capability to genetically engineer biological products with technologies such as CRISPR”.
And for good measure, “Pyongyang is capable of weaponizing BW agents with unconventional systems such as sprayers and poison pen injection devices”.
The same report raised concerns about China’s compliance with the Biological Weapons Convention, pointing to how “PRC military medical institutions conduct toxin and biotechnology research and development with potential BW applications” and that there is “no available information” to suggest that China has destroyed items associated with its historic offensive bioweapons programme.
Nation states that will not struggle to obtain nasty virus strains, won’t have to operate in sheds, and will have the ability to draw on researchers with elite training will struggle far less to weaponize biological design tools.
Closing thoughts
We’re not approaching biorisk and AI from an alarmist or doomer standpoint. We believe that there is a significant gap between something being hypothetically possible and someone being sufficiently motivated and capable of implementing it.
Do we believe a wave of AI-accelerated bioattacks is imminent? No.
Do we believe that there is any reason to slow down AI-first biology research? No.
This research has potential to do a huge amount of good for the world.
Instead, we believe that we should be making the best possible use of the time we have to design robust safety measures.
There are signs of the community coming together on some of these questions. For example, protein design researchers are committing to certain risk management standards, while leaders in the field like David Baker and George Church have outlined the need for a protein design security strategy. There’s also encouraging research that suggests traditional screening tools can be effective for picking up AI-redesigned sequences if they’re proactively patched. At Air Street Capital, we are interested in meeting teams working on biodefense and adjacent fields. We have lots of experience in AI for drug design (e.g. Recursion, Exscientia, Valence Discovery, Profluent) and believe similar capabilities can be effective for biodefense.
But much of this work remains very high-level. The Geneva Centre for Security Policy is unusual in having laid out detailed policy recommendations that could counter de novo threats. They outline measures spanning prevention, detection, and response. To prevent misuse, they recommend banning experiments that identify pandemic-capable agents, implementing universal DNA synthesis screening, introducing legal liability for disclosing harmful information, and agreeing to an international "pandemic test-ban treaty." However, this was published back in November 2022. Recent in traditional policy terms, but ancient history when it comes to BDTs.
For detection, it advocates a global early warning system using untargeted nucleic acid sequencing at travel hubs, targeted diagnostics for known pathogens, and personalized risk notifications to prevent spread.
Some of these measures may be drastic or disproportionate at the moment, but the policy debate needs to move from either high-level generalities (“someone else should do something about this”) and ludicrously extreme solutions (“pause AI”) to exploring specifics. We’re seeing signs of this work happening. For example, the UK Government is teaming up with Oxford Nanopore to test genetic samples from severe respiratory infections as part of a pandemic surveillance system (similar to previous work it shuttered).
We also believe that AI will be a big part of any potential solution. To return to Margaret Kosal: “It’s also important to recognize—and it often gets a lot less attention—that there are opportunities for AI/ML to contribute to non-proliferation efforts, including in context of protein synthesis, through the development of AI/ML capabilities to detect, deter and limit proliferation.”
If you are working on anything in this domain or exploring new research questions - however early - get in touch!