Introduction
For what feels like a long time in AI, 2-year old ElevenLabs had a pretty clear run in the voice cloning and text-to-speech market. It was up against half-hearted, underpowered offerings from big tech companies or slow, unintuitive open source models like Tortoise.
Although larger players like OpenAI built powerful text-to-speech models, they adopted slow and cautious release strategies.
It’s not difficult to understand why. With frontier labs already embroiled in a series of legal disputes around copyright, they probably didn’t want to be accused of flooding the Internet with deep fakes in the run-up to a series of globally significant elections. But this is already beginning to change.
So should start-ups like ElevenLabs be worried?
In a recent interview, Carles Reina, VP Revenue at ElevenLabs, shared an interesting perspective:
We're seeing mid-market logistics companies, hospitals, and 200-employee e-commerce companies all wanting to implement AI. They see the benefits but don't have the resources to do it themselves. This changes how I, at Eleven Labs, approach customers but also gives long-term moats, contracts, and success. Once you secure one of these non-tech customers, they're likely to stay with you - they don't want to change, unlike big tech companies that can easily switch APIs.
This touched on something important and often under-appreciated by those building in AI: disruption is actually pretty hard. Being better than the rest can compensate for not being first, but it’s not guaranteed.
This week on Air Street Press, we’re going to talk about the importance of speed, the challenge of friction and inertia, and what we can learn from this.
Being first
When we get asked what we look for in founders, we obviously mention engineering and product brilliance, but equally important is a strong dose of technical pragmatism and customer obsession. We’ve met many gifted builders who sometimes forget that category-defining businesses aren’t built solely by publishing papers reporting impressive benchmark performance in a lab setting. Indeed, as we’ve written elsewhere, good technology does not speak for itself.
While it’s possible to make your fortune off the back of a consumer app that goes viral, this is rare to capture lightning in a bottle.
Instead, the companies that succeed often have to i) convince someone to buy what they are selling; ii) regear their existing systems to integrate it; iii) potentially retrain their team; and iv) experience some short-term disruption or reduction in productivity to unlock long-term advantage.
This is possible, but it’s hard and it’s why most start-ups don’t succeed.
But it also means that if you’re first, you have a strong moat.
ElevenLabs was prepared to endure early controversy around the misuse of its product that scared off big tech labs.
Thanks to a good product, an early lead, and mindshare built through Instagram and TikTok creators, it built strong loyalty as it moved upmarket, with users at over 60% of Fortune 500 companies. It will have significantly more data than potential competitors on what users like and don’t like, which features drive growth versus which aren’t worth the investment, promising sectors and geographies, regulatory experience, and investors aligned with the vision.
Someone who’s built a good text-to-speech model in a lab, even if it’s theoretically better, will have none of these advantages. While they might be able to convince a few AI-native users who enjoy hacking things together to trial their work, convincing enterprise users with better things to do that they should switch from a product they already like is much harder.
You could make a similar argument about Synthesia, the AI-first video avatar platform, which outpaced HeyGen and Hour One, to build significant Fortune 500 uptake. This is a daunting lead for a new entrant to overcome:
There’s also a point about mindshare. Perplexity’s AI-powered search product likely isn’t yet deeply intertwined with many org’s tech stacks, but the company has invested significantly in brand, simply to drown out the voice of any potential competitor - going to lengths that few others would.
Any new AI-first search challenger has to both create a competing product, convince investors that it’s superior to a better-funded Perplexity (not to mention incumbents with the best business model ever invented) and then build consumer awareness from scratch.
Frictions
In this month’s Guide to AI we picked up on some numbers in Menlo Ventures’ (very originally named) State of Generative AI in the Enterprise Report.
One of the most striking headline results is how Llama’s enterprise market share hasn’t increased at all this year. Despite models in the Llama 3 family now being competitive with the frontier across a number of metrics, the numbers remain flat. Similarly, Mistral, which routinely reports impressive benchmark performance has seen its market share decline.
Something that’s both really good and free. What’s not to like?
But this isn’t wholly surprising. The Llama users we know tend to be in one of two categories: AI researchers or AI-first start-ups. In short, they’re sophisticated users who understand model deployment and hosting, who are prepared to accept trade-offs in simplicity for lower costs and greater customization.
That’s a valuable niche, but if you’re building at the foundation layer, you’re unlikely to become the dominant player by exclusively serving it.
Most users will likely want something more straightforward, where they can abstract away as much complexity as possible. In the past, we’ve written about how enterprises (and their legal and procurement teams) will likely be biased towards providers who can offer them familiar software license-like terms.
Switching from using Claude or an OpenAI model to Llama will objectively involve more work and some disruption. To Carles’ point at the beginning, if you’re a mid-market logistics company or a hospital - is it likely to be worth it?
But, looking at the chart - hasn’t OpenAI lost significant ground to Anthropic? Doesn’t that undercut our point on frictions?
We’d note two things.
Firstly, OpenAI and Anthropic primarily derive their revenue from different sources.
75% of OpenAI’s revenue comes from its consumer-facing product, while 85% of Anthropic’s revenue comes from its API. Menlo’s numbers don’t distinguish between the two - and the survey could well be including people who expense a ChatGPT subscription to their employer (functionally a consumer subscription) or pay for their own.
Switching between consumer chatbots is essentially a painless process. Switching from one proprietary LLM to another as an enterprise is harder, but as we’ve covered in the past, the lock-in effect is often smaller versus many other tools.
There’s a parallel dynamic in hardware, which we’ve covered before in our writing on Intel and NVIDIA. Companies looking to challenge NVIDIA’s stranglehold on the AI market have often attempted to compete on individual metrics (e.g. inference speed or performance-per-dollar and performance-per-watt). But this approach fails to understand that for a company that’s optimized its models for CUDA and whose team is accustomed to the software ecosystem, the bar for switching is incredibly high. This doesn’t even account for how NVIDIA has cornered the data center market. How many teams want to either abandon hardware they’ve bought or reconfigure a cluster they’re renting from scratch?
Disruption is hard
We’ve talked a lot about challenging high-performing incumbents. Taking on ElevenLabs or OpenAI is obviously difficult. But what about sectors where there’s been next to no innovation in recent years?
This is where inertia and lock-in take hold. Legacy tools or software can maintain a dominant market share for years after better alternatives have come along. Adobe Flash persisted to 2020, even after HTML5 provided better alternatives for web animation and interactivity, while new entrants took years to start chipping away at Oracle Database’s market share.
Perhaps one of the worst examples of this trend is in the world of Internet browsers. Internet Explorer 6 was released in 2001, bundled in with Windows XP. By the turn of the new millennium, Microsoft’s superior financial resources meant that it had convincingly beaten Netscape in the First Browser War. The combination of limited competition and Windows as a distribution channel led Microsoft to consider web browsers solved. As a result, they froze IE development, releasing no major updates between 2001 and 2006.
In this time, IE6 went from being a passable product to being an actively bad one. There was poor practice, like security vulnerabilities being left unpatched for years at a time. But, more importantly, the product did not keep up with the changing nature of the web.
From about 2004, Ajax and Web 2.0 began the shift from static web pages to interactive applications where content could update without refreshing the entire page. IE6 struggled with this new paradigm because it used non-standard ways to handle background data loading (requiring special IE6-specific code), had a slow JavaScript engine with memory leaks, and implemented web standards like CSS incorrectly.
However, new challengers like Firefox, which could both handle this shift and had modern features like tabbed browsing, gained little traction. Internet Explorer was often reported as ‘slipping’ in this period, but its market share remained comfortably over 80%.
But why?
For most consumers in 2024, the act of switching browsers seems trivial. Downloading and installing software is a routine task, while transferring over your bookmarks and passwords is usually straightforward. Literacy and confidence levels were lower 20 years ago, while many users were likely unaware that better alternatives to IE6 existed.
Meanwhile, because of its dominance, many developers had to build applications tailored for IE6’s quirks. The time and expense in switching could run into millions of dollars for a large organization. This created a network effect-driven doom loop where people built for IE6, which meant sites worked best in IE6, which caused people to continue to use IE6, even though it sucked.
Perhaps the most notorious example is ActiveX, a now deprecated Microsoft software framework that allows browsers to share data or functionality with apps (e.g. displaying and playing a video on a web page). There was a panic in South Korea in 2022 when Microsoft moved to end IE support, 7 years after deprecating the browser. This was because much of the country’s payments infrastructure, along with web services run by the public sector, were still reliant on ActiveX.
Even as the world attempted to move on from IE6, developers still had to maintain two separate codebases - one for modern browsers and a simplified version for IE6.
Meanwhile, Microsoft had to build an IE6 “compatibility mode” into later browsers, so people could access sites and web apps built for it.
But sometimes even that wasn’t enough. Darkly, you can find old Internet discussions where people need to downgrade because of this.
But of course, IE6 eventually died, as did IE more broadly.
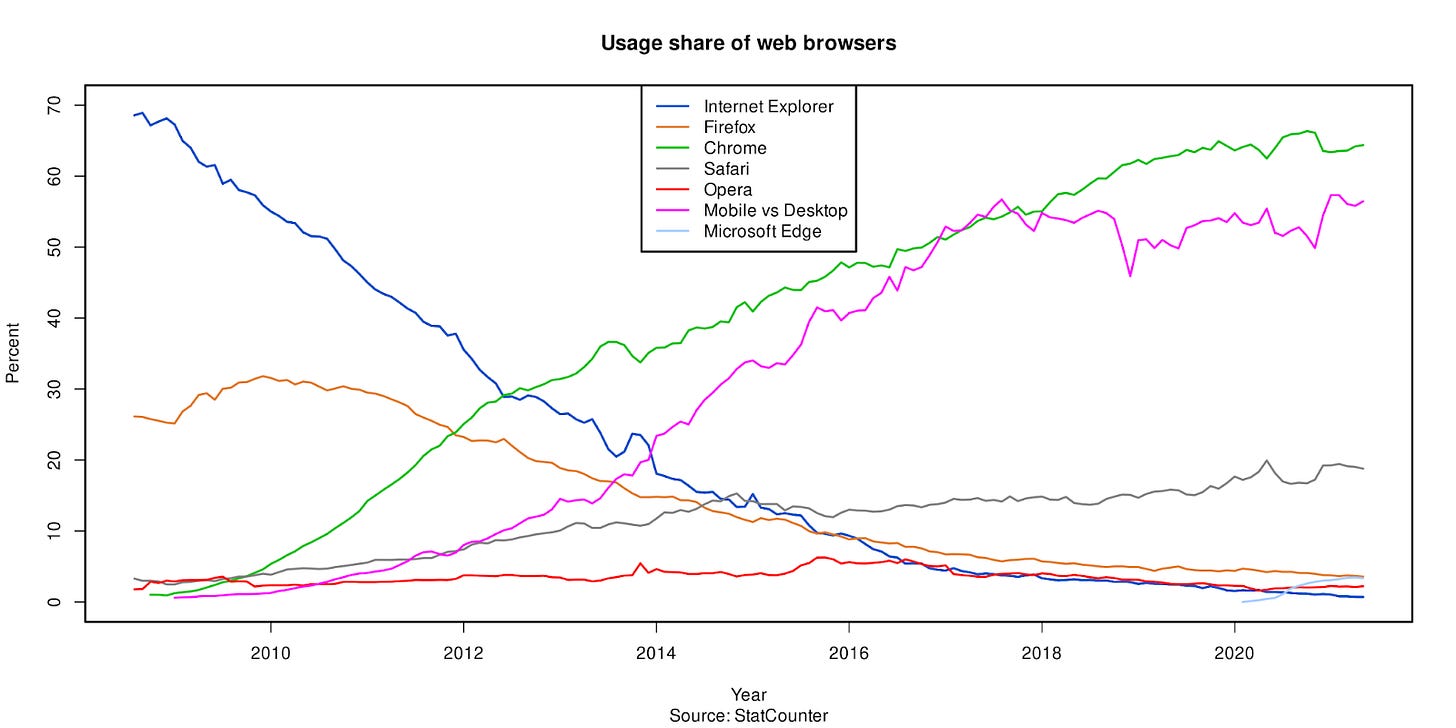
Then, Chrome launched in 2008. Not only was it paired with Google’s massive distribution, it had a decisively better ability to run web apps, as they were becoming prevalent. This made the performance difference both obvious and important. The rise of smart phones also rendered IE’s fragile desktop monopoly irrelevant, while raising user expectations about functionality.
What can we learn from this?
In short, no company is undefeatable. But even if a product is bad, if it achieves widespread enough early adoption, it can take years to displace it.
All empires eventually crumble?
If we believe that AI is going to remake everything, could it also loosen the grip of legacy software?
Yes and no.
It’s possible to imagine tools produced by companies like Vercel or Replit accelerating development and deployment optimization. Vercel’s v0, for example, could help refactor and modernize legacy code, while Replit tools like Ghostwriter could probably help document existing codebases.
AI-first tools could help reduce switching costs by accelerating the development of compatibility layers or providing better tooling for incremental migration.
Alternatively, generative software may have a role to play. Tools produced by companies like our friends at Patina Systems, might help democratize the rapid creation of software that works for them.
We also shouldn’t underestimate how modern cloud services are reducing switching costs. Many enterprise applications are now containerized - meaning different tools have their own codebase, communicate with each other through well-defined APIs, and can be deployed independently. We’re unlikely to see a whole country’s payment infrastructure depending on one company’s browser plug-in again.
But this is no cause for start-ups to become complacent. The biggest frictions in enterprise software migration often aren’t technical.
It involves changes to business processes, user training, data migration, compliance requirements, and integration with other systems. Although, we would contend that our friends at Interloom could help with some of this, by turning unstructured institutional knowledge into structured, AI-workable data.
Sticking with South Korea’s bizarre ActiveX technical debt - that stemmed from institutional dependencies, many of which were the product of bad policy, rather than no one in one of the world’s most technologically advanced countries understanding browser plug-ins.
It’s easy to see a world of smaller, more agile enterprises chopping and changing tools rapidly. But start-ups hoping to break into institutions or big enterprises will likely need to fight quickly and fight hard for some time to come. It means thinking about these challenges from day one.
Closing thoughts
At our SF State of AI launch meet-up, we were lucky to be joined by Henry Modisett, who heads design at Perplexity. He reminded us that building for normal people isn’t the same as building for AI experts. Users shouldn’t know what model a product uses (nor do they even care to know), they shouldn’t have to decode the meaning of words like “agentic”, or have to fiddle around with model settings.
If you are AI native and are only thinking about AI natives, you are unlikely to consider friction or inertia as real forces. Trying a tool for the first time or implementing a new system is a source of excitement for you. For 99% of the population, any change to technology they regularly use is likely to be a source of nervousness or dread. If you can’t show your AI product to a normal person and have them immediately grok what it’s about, you’re in for some heartache. Getting them to try once might be doable, but invoking repeat usage or a full product switch for the same job becomes a reach.
We are currently in an environment where, thanks to the speed of frontier model development, there’s currently a big gap between our current capabilities and the products we’ve managed to build. Instead of trying to build the next incremental improvement on something that already exists, only to hit up against a wall of inertia, think about the delightful products you can build now that will generate quality data for you to build models native to the problems your users have.
If you think you’re building something that’s ‘first’ or are helping to break the vice-like grip of a legacy tool - get in touch!